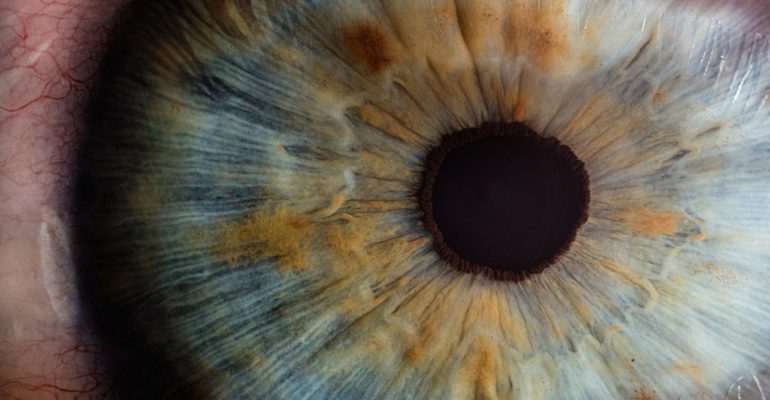
Deep-Learning Algorithm Shows Promise in Diabetic Eyes
Automated software accurately identifies referable retinopathy
An algorithm based on deep machine learning demonstrated high sensitivity and specificity for detecting referable diabetic retinopathy, researchers reported.
In two validation sets (EyePACS-1 and Messidor-2) at the operating point selected for high specificity, the algorithm had 90.3% and 87% sensitivity, and 98.1% and 98.5% specificity, for detecting referable diabetic retinopathy, which was defined as moderate or worse diabetic retinopathy or referable macular edema, according to Varun Gulshan, PhD, of Google Inc. in Mountain View, Calif., and colleagues.
The EyePACS-1 algorithm had a receiver operating curve (ROC) of 0.991 (95% CI 0.988-0.993), while the algorithm of the Messidor-2 cohort had a ROC of 0.990 (0.986-0.995), they wrote in the Journal of the American Medical Association.
The authors said they wanted to determine how the performance of an automated deep-learning algorithm would compare with manual grading by ophthalmologists for identifying diabetic retinopathy in retinal fundus photographs.
“Google has been working on computer vision for a while, and we use this technology in many of our products. For example, deep-learning in ‘Google Photos’ enables users to search their image library for things like ‘dogs’ and ‘hugs,'” explained co-author Lily Peng, MD, PhD, also of Google, to MedPage Today.
“Images are commonly used in health and medicine, and we thought our technology might be able to help in this space,” she said. “We started working on diabetic retinopathy [DR] specifically because we saw an opportunity to provide more people with access to care. Blindness caused by DR is preventable if caught early. But today, too few people are diagnosed because they don’t have easy access to specialists who can help. In India, for example, there is a shortage of ophthalmologists and optometrists; one study in Indiafound almost half of DR patients already presented with loss of vision.”
For the current research, a total of 128,175 retinal fundus photographs from patients in the U.S. and India were graded on a scale from moderate to worse diabetic retinopathy, referable diabetic macular edema, or both. Each image was then graded between three to seven times by a volunteer group of ophthalmologists, used as the basis for the creation of the deep-learning algorithm. The training process for the machine involved comparing the trained severity grade against the grade given by the panel of ophthalmologists, and repeating several times over for accuracy.
The software had some limitations, the authors noted, specifically that the reference standard used for the study was the majority decision of all ophthalmologist graders, so the algorithm may not perform as well in images with subtle findings that even ophthalmologists would not identify.
In an accompany editorial, Wong Tien Yin, MD, PhD, of the Singapore National Eye Centre, and Neil Bressler, MD, of John Hopkins University in Baltimore, applauded this use of technology for tackling the “public health care problem” that is diabetic retinopathy, which affects over as estimated 100 million individuals across the world.
Despite guidelines recommending screening for diabetic retinopathy, there generally is a lack of resources in order to screen the estimated 32 million individuals each year at risk, according to the editorialists. Computer software can “lead to improved patient care and outcomes,” although they pointed out that there will be challenges to widespread clinical implementation.
“How does such software ‘fit’ in a clinical system?,” they wrote. “Should the software be incorporated into retinal cameras and thus used at the point of care by ophthalmologists, optometrists, or other health care professionals?… Alternatively, should centralized diabetic retinopathy reading centers be established in the United States and … in low-resource settings where few ophthalmologists are available to care for all of the patients with diabetes?”
Wong and Bressler suggested that additional studies to further validate the algorithm are required, specifically to compare severe diabetic retinopathy to referable diabetic retinopathy, which the study mainly focused on. Also, the current algorithm may not be ready to fully replace manual screening because it was only trained to identify diabetic retinopathy, and not other conditions such as glaucoma, and macular degeneration.
Gulshan’s group said they are continuing to test the algorithm for further validation. “We have been working closely with leading eye hospitals in India … to study the algorithm in clinical studies. The data so far is encouraging, and we look forward to sharing these results in publications soon,” Peng said.
The study was funded by Google.
Gulshan, Peng, and some co-authors disclosed a patent pending on processing fundus images using machine learning models. One co-author disclosed relevant relationships with Google and the California Health Care Foundation.
The editorial was funded by the Johns Hopkins University.
Yin disclosed a patent on automated diabetic retinopathy screening software and relevant relationships with Abbott, Novartis, Pfizer, Allergan, and Bayer. Bressler disclosed a patent on a system and method for automated detection of age-related macular degeneration and other retinal abnormalities.
- Reviewed by Robert Jasmer, MD Associate Clinical Professor of Medicine, University of California, San Francisco and Dorothy Caputo, MA, BSN, RN, Nurse Planner
Primary Source
Journal of the American Medical Association
Secondary Source
Journal of the American Medical Association